New method reveals regime shifts in future ocean CO2-absorption
The amount of CO2 absorbed by the ocean varies from year to year. New machine learning techniques can help estimate the drivers of these variations more precisely and thus advance our understanding of how climate change may evolve in the future.
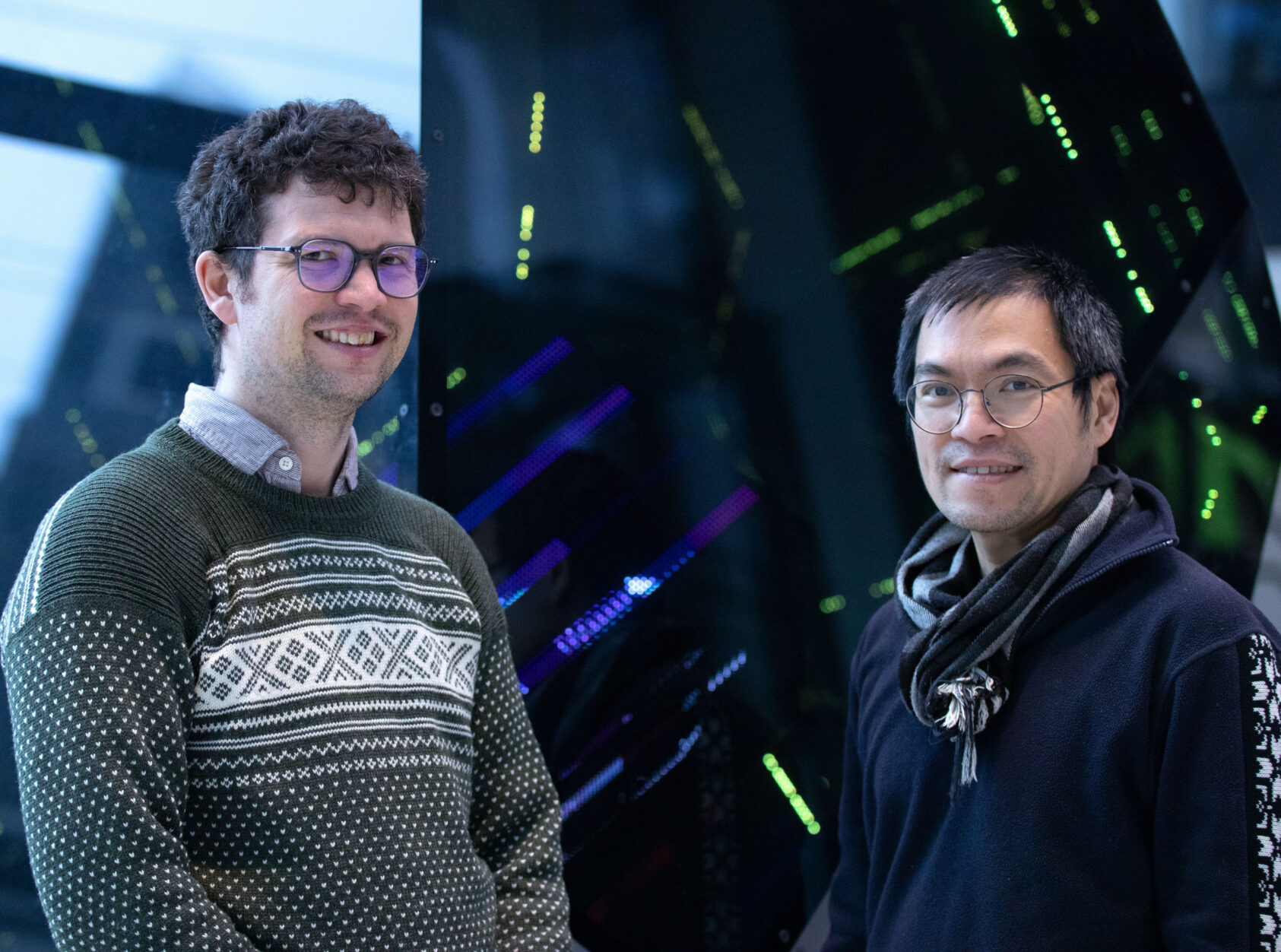
Source:
Thomas Hovmøller Ris
Damien Couespel and Jerry Tjiputra
The ocean absorbs around 25% of carbon emissions from human activity each year. The quantity of carbon absorbed by the ocean is removed from the atmosphere. This has a significant impact on global warming pathways and thus helps limit climate change. However, we don’t fully understand how this works. Estimates of the amount of carbon being absorbed by the ocean vary from year to year.
– The variation from year to year can reach 20% of the average uptake in areas like the equatorial pacific and Southern Ocean. This variation is driven by the natural variability of the Earth’s climate and can be amplified by climate change. For example, we know that during El Niño years, the CO2 absorption is usually stronger. But in many other areas, such as the Pacific and the North Atlantic, we know very little of the drivers, researcher Damien Couespel explains.
– In these areas we need a detailed understanding so we can better predict future climate change as well as detect and attribute human-induced changes to the climate, Couespel adds.
Machine learning method might be a solution
Damien Couespel is one of five climate researchers from NORCE Norwegian Research Centre and Bjerknes Centre for Climate Research who recently published a paper in the well-known and respected journal from the Nature portfolio, Communications Earth & Environment. The four other NORCE researchers are Jerry Tjiputra, Klaus Johannsen, Pradeebane Vaittinada Ayar and Bjørnar Jensen.
The exact drivers for variations in ocean CO2-absorption in different parts of the ocean are still debated among scientists. In search of a better tool for understanding the drivers, the five NORCE researchers used novel machine learning techniques.
– When you simulate the role of the ocean in climate change with state-of-the-art climate models, such as the Norwegian Earth System Model (NorESM), it requires a lot of computing power. If we want to test the impact of one driver, it will usually require us to run the full climate model. And we want to test many drivers, in several models and with multiple scenarios of climate change, which quickly increase the computational and human resources tremendously, Couespel says.
– Moreover, the quantity of data is so large that it requires a lot of time to analyze them. This is where machine learning techniques come in. They are powerful tools for identifying relations between quantities so they can help us to efficiently explore large volumes of data from climate models and observations.
Identified key drivers for variations
The machine learning techniques gave the researchers a possibility to test how features like sea surface temperature, sea ice concentration, mixed layer depth, and chemical composition contribute to variations in carbon absorption by the ocean.
In the study, Couespel and colleagues reconstructed the variations in carbon absorption from these features using the machine learning algorithm. Afterwards they validated it with the original data from the climate models.
– The strong correlation between our reconstruction and the original data indicates that we can accurately reproduce most of the year-to-year variability in CO2 absorption with this technique.
They were then able to test the importance of each feature in the variability of CO2 uptake much more quickly than using the conventional approach. The test revealed that alkalinity and dissolved inorganic carbon (DIC) are the key drivers of variation in CO2 absorption.
– These findings are aligned with the current knowledge and show that the reconstruction by the machine learning algorithm is meaningful. And we also show that in most of the ocean, the role of DIC in the future year-to-year variability of CO2 absorption may be reduced. This extends similar findings from the equatorial pacific to the rest of the ocean, Couespel concludes and adds:
– Of course further work is required to strengthen our results but the study shows what other climate researchers should pay attention to, in order to better predict variations in ocean CO2-absorption, and even how machine learning could be used to produce new knowledge.
With the growing number of model simulations and the output they produce, machine learning could play an increasing role in advancing our understanding of how climate change may evolve in the future, Couespel believes.